We published a paper on theory of predictive representations in hippocampus and entorhinal cortex and how these representations help hippocampus support quick and flexible planning. It is a pretty good paper in a lot of ways, so if any of those words appeal to you, you should check it out! Here is a link to the full text without paywall (I think):
The hippocampus as a predictive map.
KL Stachenfeld, MM Botvinick, SJ Gershman.
Nature Neuroscience (2017).
View on readcube.
We also wrote a blog post about it (with some help from the DeepMind coms team) if you want a more approachable version.
Basically the main idea is that hippocampus is representing current position as a blur of present and future location. This sacrifices some information about exactly where you are and exactly where certain sequences of actions will take you next. But it let’s you very quickly compute stats like “on average, how much reward will I get in the future from this starting position?” without having to recompute all the stats from scratch when reward moves around. That stat ends up being a pretty major one for humans and animals seeking joy in life, and the necessity of rapidly computing this stat forms the normative backbone of the theory.
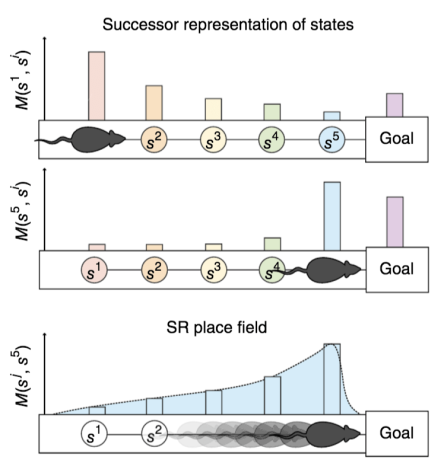
We also talk about representation compression in entorhinal cortex, and have a model of entorhinal cortex performing dimensionality reduction on the hippocampal representations. This means entorhinal cortex has to get rid of some of the information in hippocampus, forcing it to keep only the most important information. Dimensionality reduction has a lot of potential benefits, and in the paper we focus on how it can smooth and stabilize representations in hippocampus.
And the model fits a bunch of data, makes some predictions, etc.